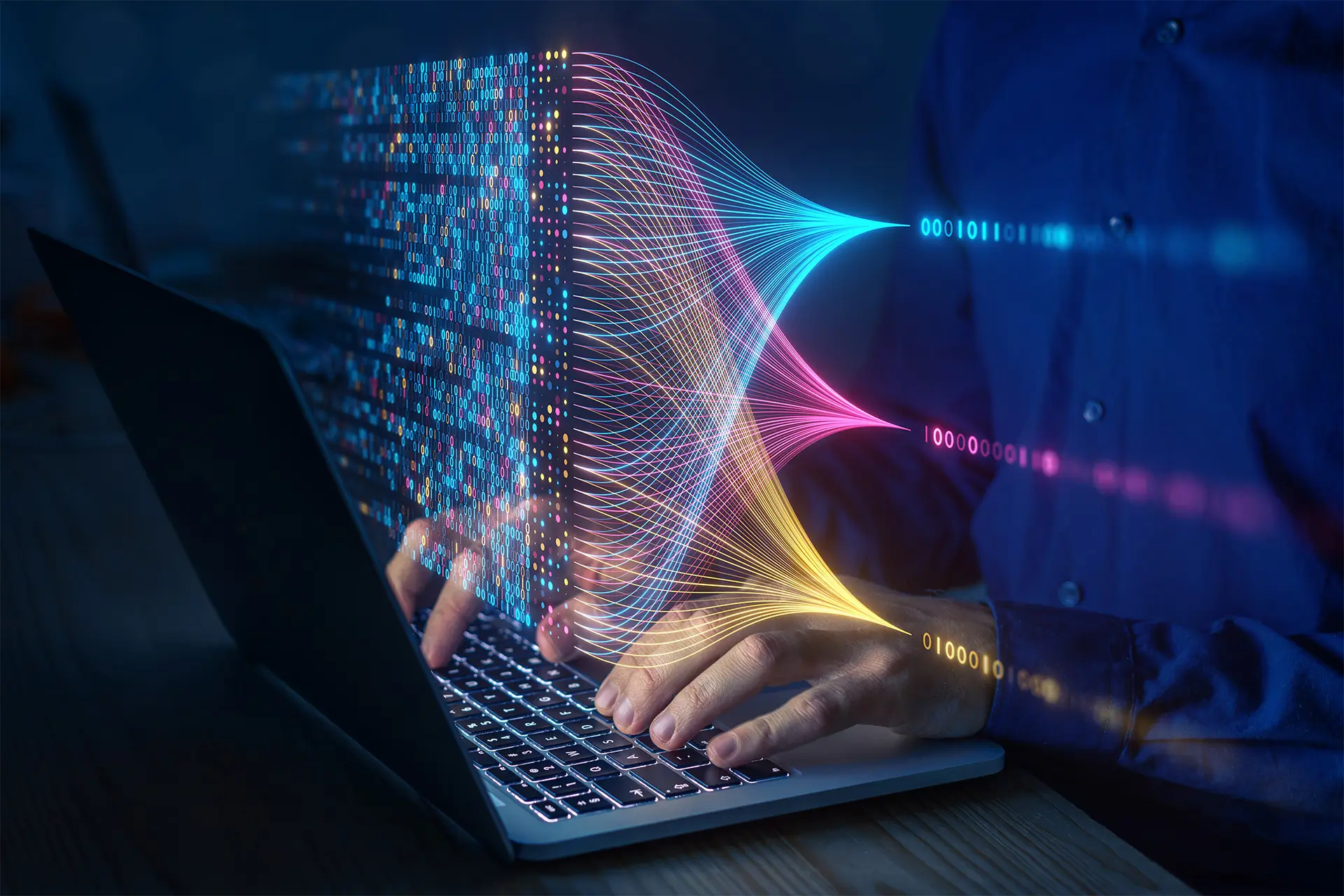
The following is adapted from Guaranteed Analytics.
Analytics is the process of turning data (unchangeable facts) into information (a story) and from there into insight. Insight is the meat of analytics because that’s where you solve business problems, answer puzzling questions, or learn something previously unknown to propel your company forward.
Turning information into insight is where the art and science of analytics meet. But it takes some finesse and planning. To get the most insight out of your analytics information, consider these revelations.
Information in Context Yields Insight
Imagine your utility company has an unpaid bill from a local business. Your policy is that all unpaid bills after a certain date result in termination of service until the account is paid in full.
Now, if you took that one unpaid bill and cut off the customer’s service you’d be following your protocols… but you might also be hurting your company in the process. Why? The local business with the unpaid account is part of a chain of restaurants that have 10 outlets all served by you.
If you’re studying information about unpaid bills in context (all accounts under the same owner, not just one isolated bill), you’d realize that all the other accounts had paid their bills in full--the unpaid one must be a fluke. Furthermore, these restaurants represent a significant source of income for your utility company, in an area where customers have a choice of utilities.
You must look at the information in a holistic enough way to get something from it, or it’s no better than raw data. The patterns and stories in the information that come from data are the stepping stones to insight. When you set up your data analysis, you not only have to ask the right questions; you need to study the results in various frameworks to see if they make sense.
Different Users Seek Different Insights
Unless you’re a tiny business, you can’t set up an analytics system and expect it to function the same way for everyone. One size fits all could make your analytics program DOA.
When deciding what information to give to various end-users in your company, you need to think about things like their technical ability, their experience drawing conclusions from analytics information, and what they need to perform their jobs. User types typically fall into three segments: knowledge workers, super users and report builders, and data scientists.
Knowledge workers, who make up the majority of information users, are an important conduit for driving analytics ROI. Deliver information to them in a guided environment that empowers them to use it easily.
Superusers and report builders generally need more granular data and may roll it into reports for knowledge workers. Data scientists make up a small percentage of users. They typically develop their own data mandates independent of executives. They identify opportunities found in information but don’t act on them.
Ignore the Tails and Find the Gold in the Middle
Most businesses know their best and worst merchandise performers, their highest executing employees and those who are giving them headaches. That’s the obvious stuff, and it’s a feel-good waste of time.
To make the most of your analytics information, you need to shoot for the middle, where the mystery is. Political users of analytics do this very well. They know time isn’t well spent on the extreme ends of the spectrum, so they focus in the center.
Imagine you sell plumbing supplies. You know which pipe is doing so well it’s often back-ordered. And you know which parts you’ve had to mark down twice to move off the shelves.
How are you going to monetize the inventory in the middle? You might discover through your information that swimming pool contractors use a particular part, and you can market to that customer more, for example. Doing the work pays off because the middle of your inventory is where the bulk of your revenue comes from.
Slice Information into the Most Relevant Recency and Time Frequency Usability
Most analytics information is going to be used in time-sliced increments. Time-sliced data are easier to understand because they have a clear beginning and end. The facts are less ambiguous, so there’s less arguing about their interpretation.
Occasionally, you will need real-time information, such as if you need to monitor the gauges in a nuclear power plant. However, the bulk of information is better if it’s not in real-time.
Unless you’re an air traffic controller, you don’t need to know how many flights are in the air now (which constantly changes); as an airline owner, though, you want to know things like how many seats were empty on yesterday’s flights or which days of the week are busiest between New York and Los Angeles.
There’s so much insight to be gleaned from your information if you structure your quest properly. Use the gems above to help uncover hidden opportunities and answer questions for your business, and you can use those successes to springboard to more as you grow.
For more advice on getting more insight out of your analytics, you can find Guaranteed Analytics on Amazon.
Jim Rushton began his career in analytics working with some of the biggest consulting companies in the world, including Accenture, Deloitte Consulting, and IBM Global Services. Jim then moved to an executive position with Verizon, where he oversaw the company’s customer and marketing information. Leveraging his experience across corporate America, he helped found Armeta Analytics, and in the past decade, his team has helped dozens of Fortune 1000 companies learn how to monetize their data.